Banks Must Fight Financial Crime Better
& Machine Learning Provides
the Solutions
Fraudsters are growing more sophisticated, and traditional methods to fight financial crime simply don't cut it anymore. Strategically applying machine learning allows banks to thwart financial crime better than competitors and avoid a slew of costs and consequences.
Defend Your Bank Against Fraud
95% of Anti-Money Laundering Executives Prioritize Innovative Fraud Detection Solutions
Research shows that 95% of AML executives considered it a top priority to use innovative solutions to enhance fraud detection and AML compliance, such as machine learning, [AI], and cloud-based platforms.
Act Now to Tackle Escalating Financial Crime Rates
Money Laundering Reaches Up to $2 Trillion Annually
Limitations of Traditional Systems
Rules-based systems struggle to keep up with sophisticated fraudsters, resulting in high false-positive rates and inefficiencies that increase operational and regulatory risks.
Machine Learning Enhancements
Machine learning can significantly improve fraud detection by enhancing existing systems, reducing false positives, and prioritizing alerts for more proactive and effective detection.
Quick Implementation With Dataiku
Diverse Use Cases and Proven Success
“If you start with a BI tool, then you have to do all kinds of work to set up a new environment once the project progresses. Dataiku allows us to start out with relatively simple insights questions and grow toward a more specific predictive question, developing a model all in the same tool.”
Roel Dirks
Product Manager Big Data Lab, Rabobank“Recently we were working with one of the international banks. They have been developing a fraud detection system, but unfortunately, the fraudsters [were] gaming the system very quickly. So the models used to take — from building that model, validating that model, then putting it into production — the turnaround time was about 6-8 weeks. ... That's where we combine with platforms like Dataiku to build a system where you can deploy the models in days, not weeks.”
Subhadip Roy
Head of Machine Learning Engineering, AI, and Data, Deloitte“Innovating with AI is a competitive necessity — Rabobank isn’t the only one with lots of data and use cases.”
Roel Dirks
Product Manager Big Data Lab, Rabobank“The estimated amount of money laundered globally in one year is 2%−5% of the global GDP, or in the range of $800 billion to $2 trillion.”
Anti-Money Laundering Preparedness Survey Report, Deloitte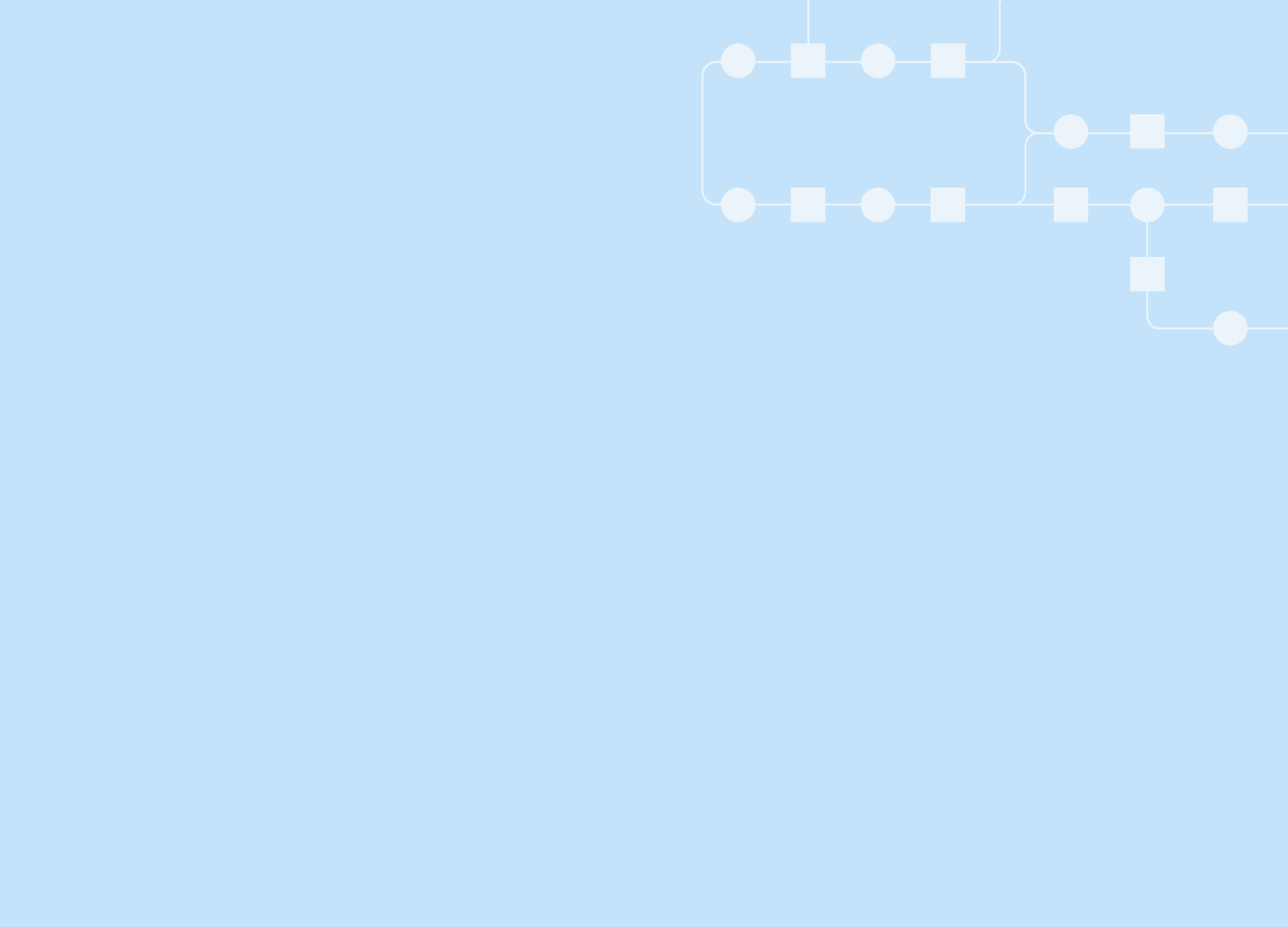